SBI-ACR BREAST IMAGING SYMPOSIUM 2023
Jiye G. Kim, PhD
Purpose
AI has transformed computer-aided tools in mammography, including FDA cleared devices for digital breast tomosynthesis (DBT). Though overall performance is often shared publicly, there has been little published evidence that AI for DBT is helpful to radiologists with different degrees of specialization and across women of different races and ethnicities. We sought to evaluate if AI for DBT could improve reader performance across readers of varying specialization (general radiologists and breast imaging specialists) and across different racial/ethnic subgroups of patients (Asian, Black/African American, Hispanic, and White).
Materials and Methods
Following an IRB approved protocol, a fully balanced reader study was conducted to evaluate if a novel custom-built categorical AI for DBT improved the performance of 18 MQSA qualified radiologists with an average of 15 years of experience (min = 6, max = 38, SD = 7.6 yrs). Readers interpreted 240 retrospectively-collected screening DBT exams (100 cancer and 140 non-cancer) once with and once without the aid of AI. Reader performance improvement was evaluated by comparing the area under the receiver operating characteristic curve (AUC) when reading with versus reading without AI. The change in reader performance was quantified across different reader types (general radiologists, n=9, defined as those who spend less than75% of their time on breast imaging and breast imaging specialists, n=9) and across exams from different patient racial/ethnic subgroups (Asian, n=7, Black, n=139, Hispanic, n=11, and White, n=83).
Results
Readers significantly improved their cancer detection performance when reading with AI versus without AI. The average reader AUC when unaided was 0.865 and improved to 0.925 when aided (difference of 0.06, 95%CI: 0.041, 0.079, p < 0.00001). Additionally, every reader had a higher individual AUC when reading with AI,with an average increase of 0.085 for general radiologists and 0.046 for breast imaging specialists. Readers also showed increased detection performance when using AI on exams from all racial/ethnic subgroups with an average AUC increase of 0.050, 0.068, 0.060, and 0.069 for Asian, Black/African American, Hispanic, and White women respectively.
Conclusion
Categorical AI helped both general radiologists and breast imaging specialists improve cancer detection performance overall, as well as within all racial/ethnic subgroups.
Clinical Relevance
Categorical AI can help general radiologists and breast imaging specialists detect cancers on DBT screening mammograms for women of all races and ethnicity.
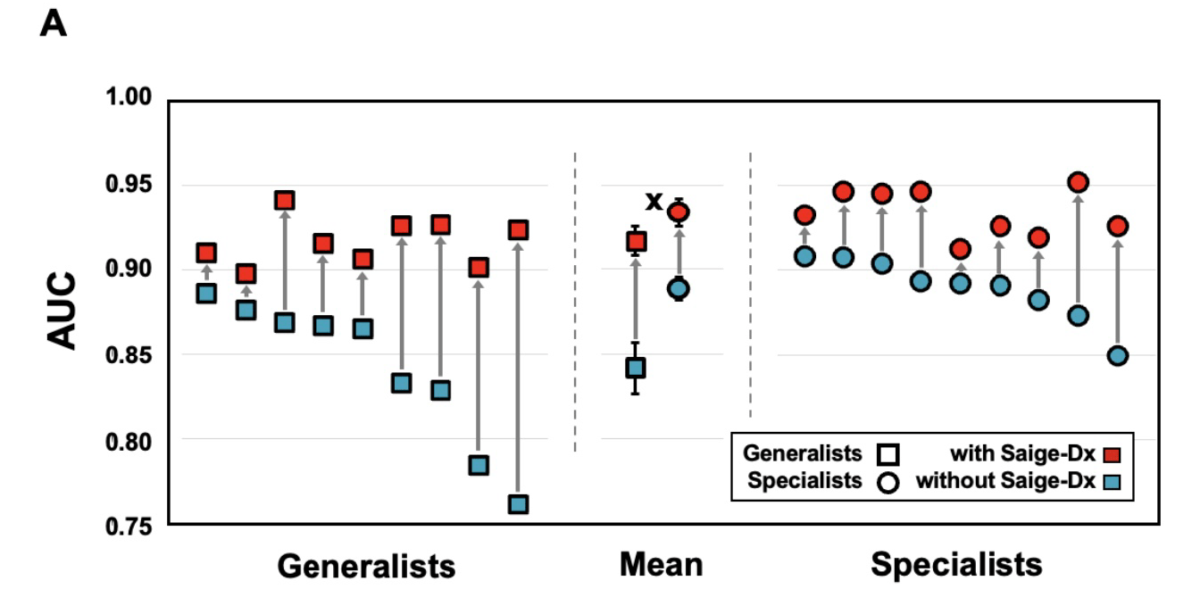