SBI-ACR BREAST IMAGING SYMPOSIUM 2023
Mireille Aujero, MD
Purpose
Numerous studies have documented that all human observers have non-zero rates of missing findings on mammograms. A goal of quality-assurance programs is to help radiologists improve their skills through peer review and feedback. In a community practice, a mechanism to improve cancer detection was investigated by implementing an exploratory AI algorithm designed to select the most suspicious exams for a second, quality-assurance interpretation. The purpose of this study was to examine the potential effectiveness of this AI-driven quality-assurance process as measured by additional cancers detected during the pilot year.
Materials and Methods
A custom-built AI algorithm that automatically identifies exams for concurrent quality-assurance peer review was implemented across a single group practice covering eight clinical sites in Delaware from July 2021 to June 2022. Performance metrics from 40,532 screening mammograms interpreted by six radiologists were collected, including the number of cancers detected, cancer detection rates (CDR), abnormal interpretation rates (AIR), and PPV1, without and with the implementation of an AI-driven peer review process. The six radiologists had a wide range of experience (from < 5 years to > 25 years in practice), and each radiologist read at least 500 screening mammograms during the pilot year.
Results
The AI-driven peer review process led to detection of an additional 41 cancers that would have otherwise been missed, in addition to the 228 cancers that were found via initial interpretations. Across six radiologists, the CDR increased from 5.3 to 6.5 (p < 0.05) with the AI-driven peer-review. The radiologists also demonstrated increased AIR from 7.7% to 8.0% (p < 0.05), however, the PPV1 of the additional recalled exams was 32.8%, which is significantly higher than the PPV1 without AI-driven peer-review (7.0%), suggesting that there is a greater fraction of cancers within the additional recalled exams.
Conclusion
AI-driven selection of exams for concurrent peer review increased the number of cancers detected for every radiologist at a small increase in AIR in a real-world, high-volume community practice. These results suggest a combination of modern, deep-learning AI plus peer review is practical and can find more cancers while providing real-time radiologist feedback leading to higher performance.
Clinical Relevance
AI can be used productively to select exams for quality-assessment peer review, leading to improved performance of all practicing radiologists while providing an opportunity for peer learning.
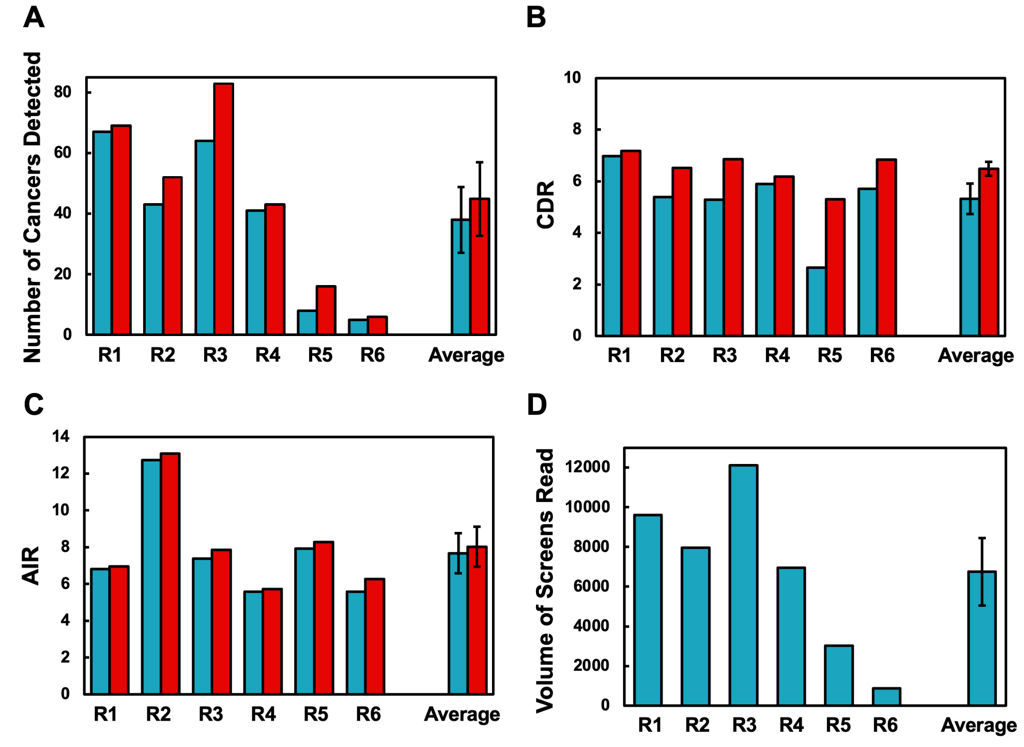